Nuclear webinar: Mingda Li
February 8, 2023
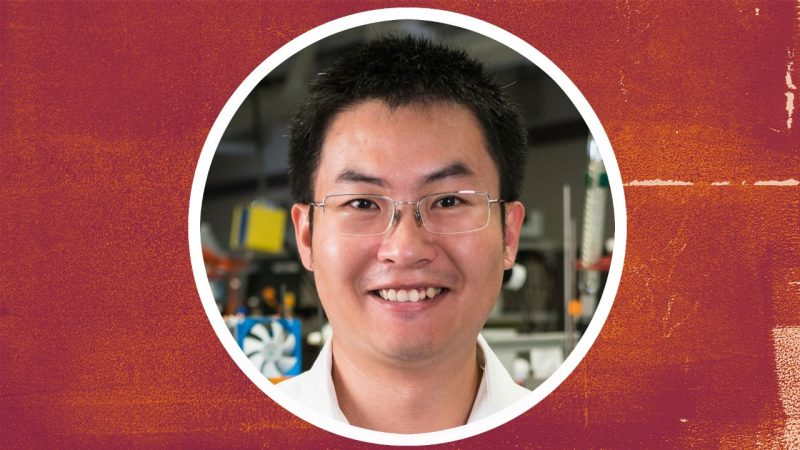
Machine learning on neutron, X-ray, and electron radiation probes for materials research
with Mingda Li, Class of 1947 Career Development Professor and Associate Professor of Nuclear Science and Engineering, MIT
Feb 17, 2023 10:10 AM
Modern radiation probes like neutron, X-ray, and electron beams have evolved into rapidly growing fields for material properties’ detection. With improved controllability and increased brightness, radiation probes are generating more data than ever and their interpretation could be a bottleneck for studying next-generation materials that could be of critical importance for nuclear industry and beyond.
In this seminar, we showcase how machine learning could address the challenges of radiation-based materials measurements from a multi-facet perspective. As a starter, we show that radiation detection can be improved with fewer pixels if machine learning is applied to the detector array readings for directional mapping and radioactive source localization [1]. Second, we show that by transforming the neutron data from original space to a latent space, it can reach super-resolution to better resolve some nuanced effect in materials [2]. Third, given the importance of thermal transport in nuclear engineering, we show that it is possible to learn the hidden thermal transport in materials with unprecedented details, if integrating machine learning with experiments [3]. Finally, since alloys are crucial for nuclear structural materials, we show that it is possible to use machine learning to learn the alloy phonon structures directly, which can be considered challenging to compute through conventional approaches [4]. We highlight the importance of the representations and envision a few problems in radiation science that can benefit from machine learning [5].
[1] To be posted on arXiv by the Seminar date.
[2] N Andrejevic et al, "Elucidating proximity magnetism through polarized neutron reflectometry and machine learning", App. Phys. Rev. 9, 011421 (2022).
[3] Z Chen et al., "Panoramic mapping of phonon transport from ultrafast electron diffraction and machine learning", Advanced Materials 2206997 (2022).
[4] https://arxiv.org/abs/2301.02197 (Under Revision)
[5] Machine Learning on Neutron and X-Ray Scattering", Chem. Phys. Rev. 2, 031301 (2021)
Mingda Li is the Class of 1947 Career Development Professor and an Associate Professor of Nuclear Science and Engineering at MIT. He completed his BS in Engineering Physics at Tsinghua University in 2009, PhD in MIT Nuclear Science and Engineering in 2015, and postdoc at MIT Mechanical Engineering. He joined back the department as an Assistant Professor in 2018. His research includes new metrology design of quantum materials, disordered materials, and thermal transport process.